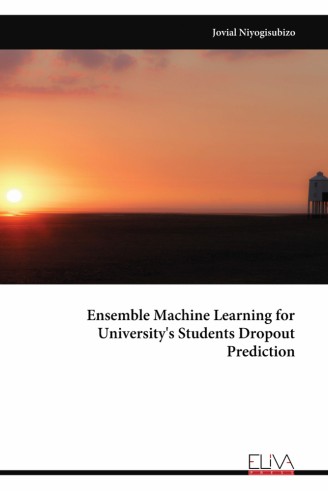
Ensemble Machine Learning for University's Students Dropout Prediction
$ 42.5
Description
Student dropout is a global concern, impacting individuals, educational institutions, families, and society. In the era of big data, accurate prediction of student dropout has become a pressing research topic. While previous studies have largely focused on specific education levels, such as primary, middle school, or university, there's a lack of research on ensemble learning approaches, particularly in predicting university student dropout using rare datasets. In this paper, we introduce a novel stacking ensemble method that combines Random Forest (RF), Extreme Gradient Boosting (XGBoost), Gradient Boosting (GB), and Feed-forward Neural Networks (FNN) to predict student dropout in university courses using a dataset from Constantine the Philosopher University in Nitra. Our method outperforms base models, achieving higher testing accuracy and superior area under the curve (AUC) evaluation metrics. The significance of this study lies in its ability to identify at-risk students and influential factors. Accurate predictions motivate students to stay committed to their studies, while educational authorities can make informed decisions about student performance. This research enhances the accuracy of university student dropout prediction, reducing the risk of course abandonment and its impact on student outcomes. Even with a small dataset, selected indicators can be valuable when various performance metrics are evaluated. The predictive models use data related to students' online learning activities and partial achievements, making the methodology reliable for predicting course completion. The results from our method are instrumental in reducing the dropout rate by identifying at-risk students and influential factors. Education agents can collaborate to eradicate the issue, focusing on learner motivation strategies to improve performance and program completion. Future research avenues include exploring deep learning and other hybrid models for student dropout prediction and considering additional influential factors not covered in this study. A feature analysis study can further assist education agents in effectively addressing student dropout challenges.