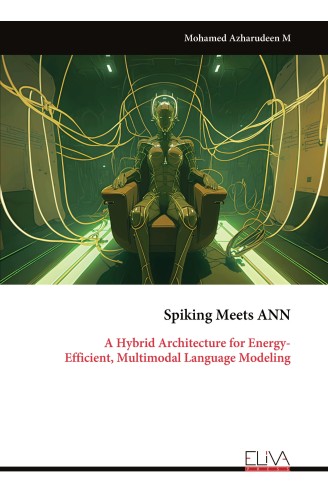
Spiking Meets ANN
$ 45.5
Description
In this groundbreaking work, Mohamed Azharudeen M. introduces a novel Spiking Transformer architecture that fuses Spiking Neural Networks (SNNs) with modern transformer-based language models, creating a hybrid AI capable of multimodal text generation with unprecedented energy efficiency. By replacing traditional self-attention with a spike-driven recurrent mechanism (Spiking RWKV) and integrating CLIP embeddings for vision-language tasks, this architecture brings the biological efficiency of spiking neurons to the power of deep learning. Through rigorous experimentation, this monograph explores the mathematical foundations, training methodologies, and performance benchmarks of the model, demonstrating its ability to generate coherent text while reducing computation through event-driven neural processing. Real-world applications span robotics, edge AI, low-power computing, and neuromorphic hardware—where energy efficiency is paramount. With a vision toward next-generation AI, this work pioneers a new paradigm in language modeling, proving that spikes and transformers can work together to build more efficient, intelligent systems.