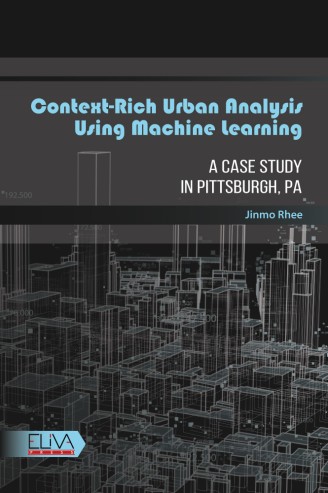
Context-rich Urban Analysis Using Machine Learning: A Case Study in Pittsburgh, PA
$ 64.5
Description
This book presents the analytic and generative potential of using machine learning methods on context-rich urban datasets. Working with a dataset of Pittsburgh's urban data, this research proposes a novel method to extract information about each building in the city in ways that reflect its immediate urban context and analyze it in terms of urban homogeneity through machine learning algorithms. The method can identify clusters of urban spaces sharing similar architectural context conditions by statistically calculating features of all building footprints and urban contexts in Pittsburgh. From the clustering results, a new type of map called a ‘datadriven map of urban morphological types’ can be generated. The interpretations and applications of this map and method will be discussed. From these discussions I will address values, meanings, and potentials of this new method in terms of architectural design, urbanism, and computation.